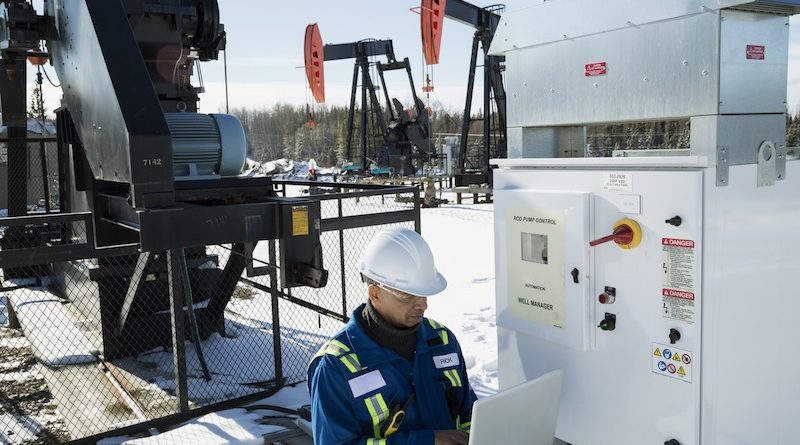
Ensuring European leadership in Artificial Intelligence (AI) for industrial applications
Artificial intelligence technologies could increase global GDP by 1 $15.7 trillion, a full 14%, by 2030 .
Industrial markets are probably the most likely to benefit from wide deployment of these new technologies.
Considering Europe has a very strong position in industry as a leader in smart manufacturing (the World Economic Forum considers that five of the top nine manufacturing “lighthouses” are in Europe), there is an opportunity for the European Union to take a leading role in the development of such emerging technologies, and their application in the industrial world.
How? By ensuring a coordinated, EU-wide approach that leverages the best innovation and investment practices from each country and breaks down current nationalised and siloed policies.
At Schneider Electric, we see four key areas where common policies and practices can help to increase investment in AI technologies in Europe.
1. Data must be easy to access and combine
The first and most critical consideration to get the most value from AI is to create a data-friendly ecosystem with integrated data models and the ability share data across different platforms.
This ensures data can be leveraged when building, using, and improving (in real time and by self-learning) AI in industrial applications.
Data scientists spend about 60 percent of their time cleaning up and organising data, and acting as “data janitors”before they can even think about analysing data and applying it to specific community problems.
If we want to enhance the contribution of data scientists in both small and large companies, which will encourage investment in AI, we need alignment on data semantics and the creation of reference models to make data compatible and easily inte- grated and aggregated to be analysed.
This alignment must take as a single EU market and, in addition, at least data models and semantics must also be aligned at an international level.
The only way businesses will invest is if it is easy for different information to be gathered and put together.
If data proves to be a “roadblock” then investment will certainly be slow, and the potential contribution of AI to GDP growth reduced.
We also believe that the emergence of multiple industrial platforms that serve data is critical to develop new applications and new solutions for advanced services using AI, particularly in industrial markets where applications and uses cases are diverse and still under development.
Such an ecosystem should be encouraged at a EU level and it should encompass big companies, small- to-medium enterprises, and start-ups to ensure a wide field for experimentation and foster the right conditions for innovation.
2. Trusted data infrastructure and policies and procedures must be implemented and monitored
Collecting, storing, and working with the type of data required to feed AI needs a high level of infrastructure, reliable power, and a cybersecure environment.
To facilitate data processing and design effective and efficient AI models, it is necessary to invest in high performance infrastructure and high-end computing systems, including the ability to run applications at the edge to reduce latency and increase reliability.
In addition, to accelerate innovative services and effective AI, trusted policies and procedures on how de-identified, geolocalised data can be collected and used must be built.
The recent General Data Pro- tection Regulation (GDPR) goes some way to developing these models of trust.
But to go further to promote investment in AI and allay concerns over data collection, use, and storage, companies must be transparent in the way that data is used and how AI models are built (as much as the technology will allow).
3. Investment in skills, training, and education
Investment in training and education is essential to develop AI and to upskill people to gain all the potential benefits offered by AI.
Training should focus on the combination of AI technologies and the development of human expertise to ensure that the best combination of both can be put to work to ensure the strong development of digital AI industrial applications.
AI represents a new way for humans and machines to work together to learn about predictive tendencies and to solve complex problems.
In industrial applications, many variables need to be factored in to achieve a successful outcome and the quality of the data that trains the AI algorithms needs to be combined with the human expertise, which is always needed for interpretation and guidance.
With the support of AI to make better operational decisions, critical factors such as safety, security, efficiency, productivity, and even profitability can be optimized.
At Schneider Electric we are fostering applications leveraging the latest AI innovations to support self-decision making by machines and processes, combined with human intervention as needed.
In the Oil & Gas industry our RealiftTM rod pump control leverages Microsoft machine learning capabilities to monitor and configure pump settings and operations remotely.
After a learning phase with experts, the AI model can detect data anomalies in temperature and pressure, for instance, and can flag potential problems.
In this scenario, intelligent edge devices can run analytics locally without having to access the cloud.
As we mimic the expert diagnostics, predictions can be easily validated, explained, and interpreted.
This means that operators are only needed onsite for identified maintenance when the application indicates that something will go wrong which can create a lot of savings when maintaining expensive, remote assets such as oil pumps.
4.The co-existence of human intelligence and AI
AI is not a direct replacement for humans and we do not anticipate that machines will replace the advanced capabilities of inter- pretation and decision making that human beings bring to industrial operations.
The co-existence of both will require a political definition to be created and regulated where ethical considerations are at the heart of any such regulation.
Not only do we need to invest in the technical skills of programmers, designers, and coders, but we also need to define the framework of their responsibilities for the outcomes of shared decision making between machines and people.
To achieve this, it is essential that private companies, academia, and governments work together to develop the understanding of the technology and its applications and define what needs to be regulated in regard to the broad usage of AI.
It’s also essential that the different skills and tools for the design, validation, control, and audit of AI models are accessible to diverse people and groups in order to provide well-rounded regulation considerations on any AI model.
In conclusion, AI brings with it the great potential for innovation to dramatically increase the productivity of industrial assets, better manage the evolution of the workforce, and achieve a more sustainable use our planet’s resources.